top of page

IMPUTATION Techniques for missing data in sepsis early prediction using time series and non-time series
Sepsis is a disease caused by an excessive immune response to infection and is considered one of the most dangerous diseases, having taken countless lives over the years. Therefore, early detection and prediction play a critical role in timely treatment. Currently, using a dataset from the intensive care unit in the United States, advanced techniques such as deep learning and time series prediction are employed to identify patients at risk of sepsis.
With the help of these cutting-edge technologies, healthcare professionals can monitor patients' vital signs such as heart rate, blood pressure, and oxygen levels, and use deep learning to analyze this data to predict whether a patient is likely to have sepsis. This makes early detection possible, which in turn can aid in timely treatment and save lives.

Predicting the Probability of Acute Kidney Injury (AKI) through
Machine Learning Methods using ICU Data
Acute Kidney Injury (AKI) is a disease that can lead to adverse outcomes and is associated with an increased incidence and mortality risk in ICU patients. It develops very rapidly and can lead to a high mortality rate within a few days. Therefore, early
diagnosis and treatment are critical in reducing mortality.
This study aims to establish a predictive model for AKI through the MIMIC-IV and MIMIC-III databases. In terms of data, pathology data after 24, 48, and 72 hours of ICU admission is used to determine whether the patient has AKI based on the
Kidney Disease: Improving Global Outcomes (KDIGO) criteria. For model construction, multiple tree-based predictive models and incremental learning methods are used for prediction, with AUROC used as the evaluation metric for
model performance.
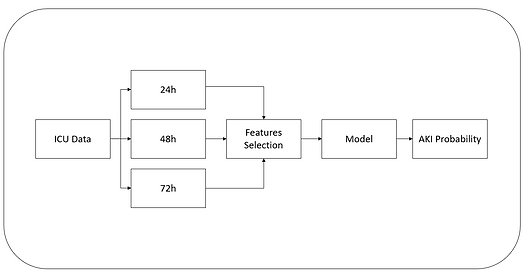
bottom of page